In issue 14 of Konzept, Deutsche Bank’s flagship research magazine, a team at the cutting edge of applying data science and artificial intelligence to stock picking explain how their research can help identify outperformers.
To understand just how wrong some financial algorithms can be, consider the ‘Hathaway effect’. In 2012, a researcher noticed that each time a film was released starring Anne Hathaway there was a jump in the share price of Warren Buffett’s Berkshire Hathaway. News articles about the actress had a similar effect on Berkshire’s stock. The likely cause of this odd relationship was algorithmic systems that traded based on keyword searches of news articles. That story neatly highlights the problems with using traditional trading algorithms to search unstructured data sources such as news and social media.
The attempted use of non-financial Environmental, social and governance (ESG) metrics in investment is not new, but as the ‘Hathaway effect’ shows, traditional systems that aggregate key words and then assign scores to stocks have severe limitations. Yet investors persist with these systems as a human simply cannot read the millions of pages of information available.
What is needed is artificial intelligence that can learn to infer context in news reports and compare with the context of a company report. That is the point of Deutsche Bank’s α-DIG tool. Using this infrastructure, data scientists train algorithms to understand context. The machines learn to prioritise certain words and phrases above others, cross reference between various sources, and translate the meaning into ‘buy’ and ‘sell’ signals.
One unexpected opportunity for machine learning and big data occurs when a stock is involved in litigation. Our models show that, on average, companies outperform their peers by two percentage points after the announcement of a litigation settlement but this does not happen immediately. That is because investors are very inefficient at digesting ESG information. Indeed, between the day before and the day after the announcement of an average litigation settlement, there is almost no stock return. Even two months after the announcement, the average stock has gained less than one per cent relative to the market. It takes a full four months for the two percentage points of outperformance to be realised.
Another big opportunity comes from cutting through the ‘greenwashing’ that is increasingly prevalent in company reports. This is where companies spin or exaggerate the green credentials of their products or processes. Consider that the amount of data reported to the SEC has increased five-fold since the financial crisis. The average number of words per quarterly SEC filing is now about 20,000, more than double the number two decades ago, and annual filings have grown to 50,000 words. No wonder that even well-informed investors have trouble sifting through the volume.
Many investors dismiss tricky-to-read non-financial disclosures but now, artificial intelligence, such as α-DIG, can finally give them the upper hand.
The article was written by Andy Moniz, Chief Data Scientist; Spyros Mesomeris, Global Head of Quantitative Strategy and QIS Research; and Luke Templeman, a thematic research strategist at Deutsche Bank Research.
α-DIG is part of dbDIG – Deutsche Bank Data Innovation Group.
In issue 14 of Konzept, Deutsche Bank’s flagship research magazine, a team at the cutting edge of applying data science and artificial intelligence to stock picking explain how their research can help identify outperformers.
Below is an extract, for the full article click here.
To understand just how wrong some financial algorithms can be, consider the ‘Hathaway effect’. In 2012, a researcher noticed that each time a film was released starring Anne Hathaway there was a jump in the share price of Warren Buffett’s Berkshire Hathaway. News articles about the actress had a similar effect on Berkshire’s stock. The likely cause of this odd relationship was algorithmic systems that traded based on keyword searches of news articles. That story neatly highlights the problems with using traditional trading algorithms to search unstructured data sources such as news and social media.
The attempted use of non-financial Environmental, social and governance (ESG) metrics in investment is not new, but as the ‘Hathaway effect’ shows, traditional systems that aggregate key words and then assign scores to stocks have severe limitations. Yet investors persist with these systems as a human simply cannot read the millions of pages of information available.
What is needed is artificial intelligence that can learn to infer context in news reports and compare with the context of a company report. That is the point of Deutsche Bank’s α-DIG tool. Using this infrastructure, data scientists train algorithms to understand context. The machines learn to prioritise certain words and phrases above others, cross reference between various sources, and translate the meaning into ‘buy’ and ‘sell’ signals.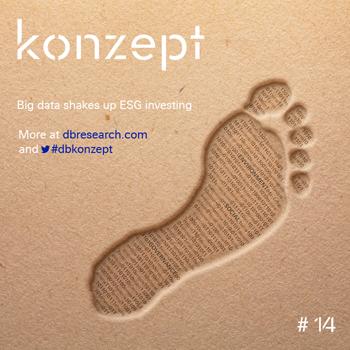
One unexpected opportunity for machine learning and big data occurs when a stock is involved in litigation. Our models show that, on average, companies outperform their peers by two percentage points after the announcement of a litigation settlement but this does not happen immediately. That is because investors are very inefficient at digesting ESG information. Indeed, between the day before and the day after the announcement of an average litigation settlement, there is almost no stock return. Even two months after the announcement, the average stock has gained less than one per cent relative to the market. It takes a full four months for the two percentage points of outperformance to be realised.
Another big opportunity comes from cutting through the ‘greenwashing’ that is increasingly prevalent in company reports. This is where companies spin or exaggerate the green credentials of their products or processes. Consider that the amount of data reported to the SEC has increased five-fold since the financial crisis. The average number of words per quarterly SEC filing is now about 20,000, more than double the number two decades ago, and annual filings have grown to 50,000 words. No wonder that even well-informed investors have trouble sifting through the volume.
Many investors dismiss tricky-to-read non-financial disclosures but now, artificial intelligence, such as α-DIG, can finally give them the upper hand.
The article was written by Andy Moniz, Chief Data Scientist; Spyros Mesomeris, Global Head of Quantitative Strategy and QIS Research; and Luke Templeman, a thematic research strategist at Deutsche Bank Research.
α-DIG is part of dbDIG – Deutsche Bank Data Innovation Group.
Further links on the topic
For more information visit dig.db.com
How helpful was this article?
Click on the stars to send a rating